Introduction
nomp: A Framework For Creating Domain Specific Compilers For C
nomp (Originally a recursive acronym: “Nomp isn’t OpenMP”) is a framework for creating domain specific compilers and a runtime or C programming language that can be used to program accelerators (GPUs, CPUs, etc.) easily and efficiently.
nomp consists of two main components: nompcc and libnomp. nompcc is the clang based compiler frontend which pre-process and compile C source files. libnomp is the loopy based runtime which can generate and tune accelerator code (i.e., kernels) from C source at runtime and then perform low level dispatch to accelerators using other high performing programming models (or backends) like OpenCL, and CUDA.
nomp uses pragmas to annotate C source with meta information about which sections of C source must be executed on accelerators. Also, pragmas can be used to perform data transfers between host (most often a CPU) and accelerators (most often a GPU). nompcc converts these pragmas to libnomp runtime calls and compile and link source code against libnomp at compile time. Then at runtime, based on runtime calls, libnomp will perform kernel generation, transformation, and execution on accelerators using loopy and other low level backends.
Instead of acting solely based on pragmas, nomp can consume a user written domain and/or kernel specific transformation scripts (written in Python using loopy API) at runtime. This gives users more control over kernel generation and execution. These external scripts don’t require any changes in original C source and can be customized and/or changed without recompiling user program. nomp also passes a dictionary to the transformations functions defined in these user scripts with information about the target accelerator and other relevant information that could help in finding optimal kernel transformations.
loopy is a code generator for array based code on accelerators. In contrast to mainstream programming models like OpenCL, CUDA, etc., which force users to make implementation choices at program logic level, loopy separates program logic from implementation details. For example, all afore-mentioned programming models force users to decide memory location for arrays (shared memory vs global memory vs registers) and mapping of loops to hardware axes at compile time. loopy provides an API for users to experiment and tune these details at runtime and thus provides a more portable way of writing kernels.
nomp architecture diagram which shows the main components and workflow is shown in the following figure.
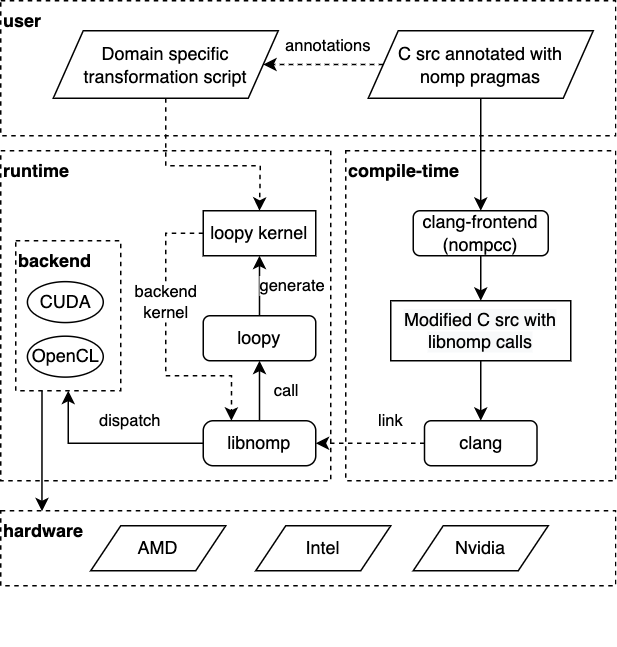
nomp combines unique features available across several mainstream programming models for programming accelerators and complement them with loopy. nomp is:
Easy to use since it uses a simple pragma based syntax like OpenMP.
High performant since it uses high performant programming models like OpenCL, CUDA, etc. for running the kernels on accelerators.
Portable since it uses loopy to separate algorithm and its final schedule on hardware. This gives users (i.e., a performance engineer) an opportunity to optimize kernels based on problem input and/or target hardware it will be executed on.
External to source code since its pragma based and uses external transformation scripts. nomp pragmas are designed to be discarded if necessary (without making any changes to the source code) and the C source code will work as expected on the CPU.
Customizable to each domain since it lets users reuse domain specific programming patterns using user defined set of annotations.
Table of Contents